New Automated Method to Segment and Measure Lymphangioleiomyomatosis Lung Cysts in CT Images
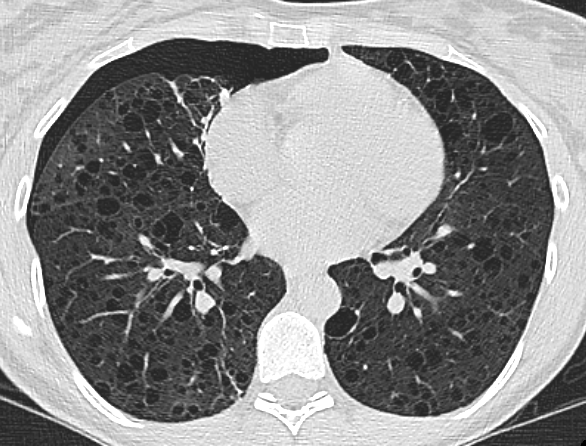
This study addresses the cystic lung destruction in Lymphangioleiomyomatosis (LAM). Because LAM may progress over many years, doctors may use pulmonary function tests, exercise tests, and CT scans to monitor disease progression and make treatment decisions. However, CT scans have been problematic due to the changing technology and varying quality of the scans over time, making it difficult to obtain consistent and accurate measurements of the lung cysts, and thus extent of disease progression.
Currently, the standard way to measure these cysts from CT scans involves semi-automated software that still needs manual adjustments by trained operators. This method is time-consuming and can be inconsistent because it relies on the operator’s skill and experience. Moreover, this procedure is impractical for long-term studies involving a large number of scans using different CT machines. To overcome these issues, we developed a fully automated method to segment and measure the lung cysts in CT images. This method uses advanced computer algorithms to identify and measure the cysts without needing manual adjustments. We tested this new method against the existing semi-automated method using CT scans taken from 24 LAM patients over 23 years, using various CT machines.
The results showed that the automated method was as consistent over time as the semi-automated method but provided more accurate cyst measurements. This was evident because the cyst scores obtained from the automated method were more strongly correlated with the patients’ lung function test results than were those obtained from the semi-automated method, which were dependent on investigator input.
In summary, this new automated method used with patients with LAM can save time and effort while providing more accurate and consistent measurements of lung cysts. This technique is beneficial for long-term studies where there is equipment turnover and could lead to better monitoring and treatment decisions for patients with LAM. We believe that this automated method could replace the current standard, making it easier to handle large-scale studies involving diverse CT scans with different manufacturers and upgrades over many years.